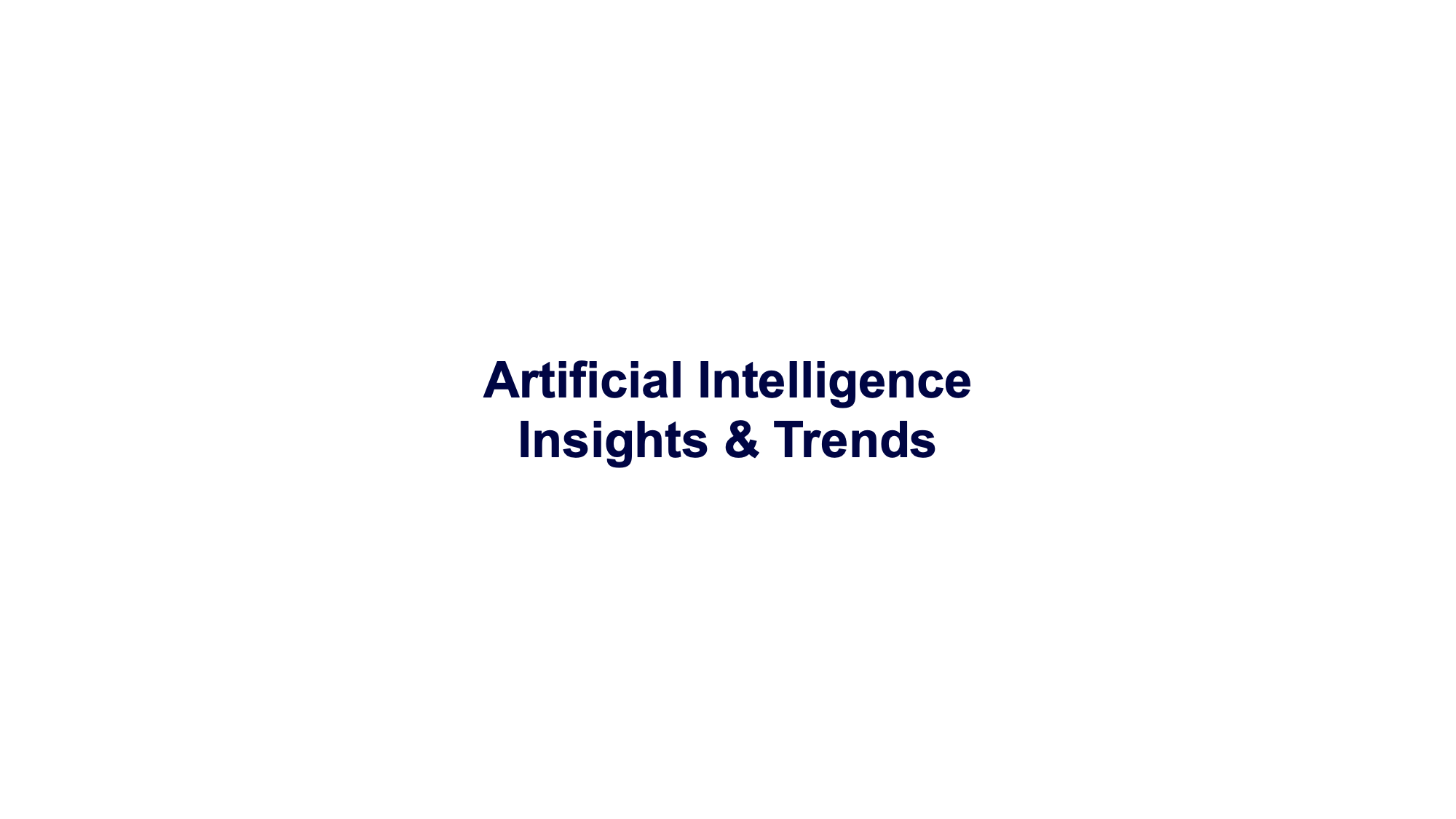
The ability to rapidly iterate and train machine learning (ML) models is key to deriving business value from ML workloads. Because ML models often have many tunable parameters (known as hyperparameters) that can influence the model’s ability to effectively learn, data scientists often use a technique known as hyperparameter optimization (HPO) to achieve the best-performing model against a certain predefined metric. Depending on the number of hyperparameters and the size of the search space, finding the best model can require thousands or even tens of thousands of training runs. Real-world problems that often require extensive HPO include image segmentation …